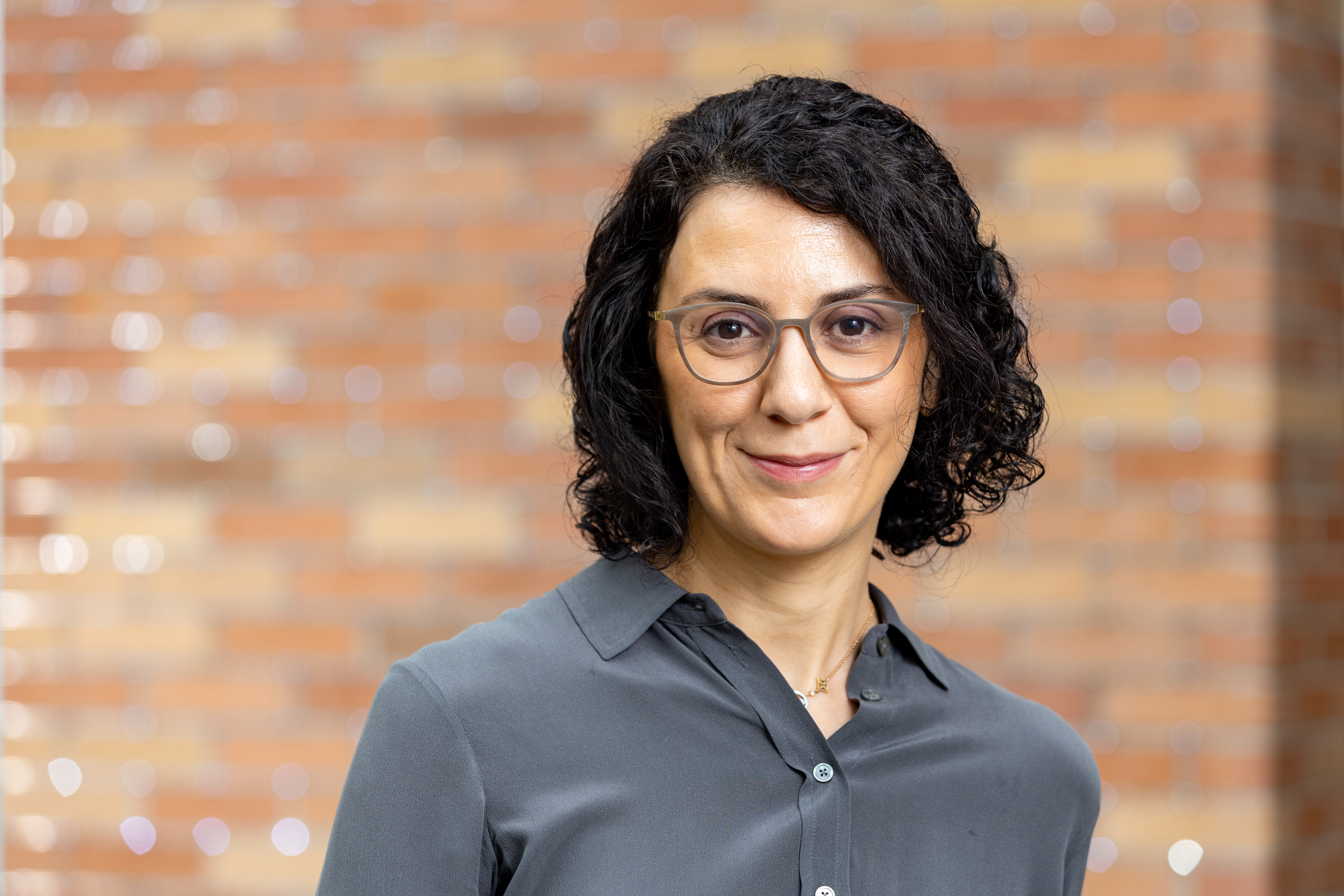
Torode Family Associate Professor
Paul G. Allen School of Computer Science and Engineering
University of Washington
Senior Director, AllenNLP
Allen Institute for AI
Email: hannaneh [at] cs [dot] washington [dot] edu
About
Hanna Hajishirzi is the Torode Family Associate Professor in the Allen School of Computer Science and Engineering at the University of Washington and a Senior Director of NLP at AI2. She received her Ph.D in Computer Science from University of Illinois at Urbana-Champaign, and spent a year as Postdoctoral associate at Disney Research and CMU. Her current research delves into various areas within NLP and AI, with a particular focus on understanding and pushing the boundaries of large language models. She has published more than 140 scientific articles in top-tier journals and conferences in ML, AI, NLP, and Computer Vision. She is a recipient of 2020 Alfred Sloan Fellowship, 2021 NSF CAREER award, 2019 Intel rising star award, 2018 Allen Distinguished Investigator award, 2023 Academic Achievement UIUC Alumni award, 2024 innovator of the year award finalist by GeekWire, and several research faculty awards from industry. The work from her lab has been nominated or received best paper awards at conferences and have been featured in a variety of magazines and newspapers including New York Times, Forbes, NPR, MIT Technology Review, Geekwire, Wired Magazine, and more.
Recent awards:
- UIUC Academic Achievement Alumni Award
- NSF CAREER award
- Sloan Fellowship
- Intel Rising Star Faculty Award
- Allen Distinguished Investigator Award
- Research faculty awards: Amazon, Facebook, Google, Samsung GRO, Bloomberg
My lab (H2lab) mainly publishes at NLP (ACL, NAACL, EMNLP), AI and ML conferences (AAAI, ICLR) across these areas:
Research
Effectively unlimited quantities of ever-changing knowledge are available online in diverse styles (e.g., news v. science text) and formats (knowledge bases, web pages, and textual documents). My research addresses the challenge of enabling rich neural symbolic comprehension and reasoning given this diversity: how can we build AI systems that comprehend and combine evidence from various and evolving sources of textual and multi-modal knowledge to make complex inferences and draw logical conclusions? We build algorithms that balance three competing desiderata: interpretable, robust with high performance, and efficient and scalable in the following categories:- General-purpose NLP. Building NLP models that go beyond solving individual tasks and can learn new tasks from their descriptions or a few examples.
- Reasoning and question answering. Building benchmarks and algorithms that offer rich natural language comprehension using open domain, multi-lingual, multi-hop, and interpretable reasoning; developing some of the first deep neural models for general reading comprehension (BiDAF), open domain QA, cross-lingual QA, multi-hop reasoning, and symbolic methods to solve math and geometry word problems.
- Knowledge acquisition from multi-modal data. Devising general high-performance algorithms to extract knowledge from textual and visual data; devloping some of the first work in extracting knowledge from scientific text.
- Representation learning. Integrating capabilities of symbolic representations into neural models to represent knowledge acquired from diverse structured and un-structured resources and forming knowledge-rich dense vectors to encode them; designing neural architectures that efficiently encode textual and visual data.
Contact
-
Office: Paul Allen Center 654
Phone: (206) 221-3921
Email: hannaneh [at] cs [dot] washington [dot] edu