About
I’m Moe, a database researcher and PhD student at the University of Washington, Seattle.
I currently work on applying of large language models to data discovery tasks such as table annotation. Previously, I worked graph compression methods, which accelerate graph analysis 10–100⨉ while introducing a minimal approximation error. I have also worked on automated machine learning (autoML), which allows for selecting performant and robust machine learning models. As well as causal inference, which aids analysts in finding true cause-and-effect relationships in complex data.
My work has found real-life applications at places from large enterprises, such as Microsoft Research, to small startups such as Virta Labs.
Recent Updates
Mar-2024 | Our “CHORUS: Foundation Models for Unified Data Discovery and Exploration” paper has been accepted to VLDB 2024 |
Jan-2024 | I’ll be on a panel on AI+DB at the NWDS 2024. |
Dec-2023 | CHORUS will be at the Table Representation Learning workshop at NeurIPS ‘23. Come say hi! |
Jun‑2023 | Read our preprint on using large language-models (LLMs) for data discovery |
Nov‑2022 | Presenting Quasi-stable coloring at VLDB 2023: try the Julia package out |
Aug‑2022 | Presented at the AutoML workshop at KDD 2022 |
Jun‑2022 | Interning at the Gray Systems Lab at Microsoft this summer, working on cardinality estimation |
Jun‑2021 | Selected as a Herbold Fellow for the year 2021 |
Jun‑2021 | Working under Chi Wang at the Data Systems Group within Microsoft Research this summer |
Aug‑2020 | View a demonstration of causal inference on relational data with CaRL, which I presented at VLDB 2020 |
Jun‑2020 | Received the Outstanding Senior Award from the Allen School of Computer Science. |
Apr‑2020 | Excited to be joining the database group at the University of Washington as a PhD student in September 2020! |
Mar‑2020 | Causal Relational Learning will be presented at SIGMOD 2020. |
Dec‑2019 | Selected as a Mary Gates Research Scholar |
Dec‑2019 | Honorable mention in the national CRA Outstanding Undergraduate Researcher Award. |
Contact
- via email: kayali @ cs dot washington dot edu.
- Twitter: @moe_kayali
- Old-fashioned mail to:
3800 E Stevens Way NE
Box 352355
Seattle, WA 98195
Profiles
Google Scholar, DBLP, Semantic Scholar, ORCiD .
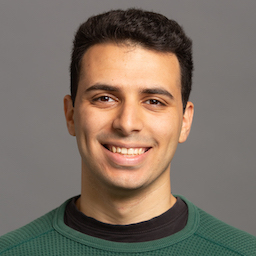
My Erdős number is 3. My Erdős–Bacon number is ∞.